Use modeled data to reach specific consumer audiences
October 31, 2024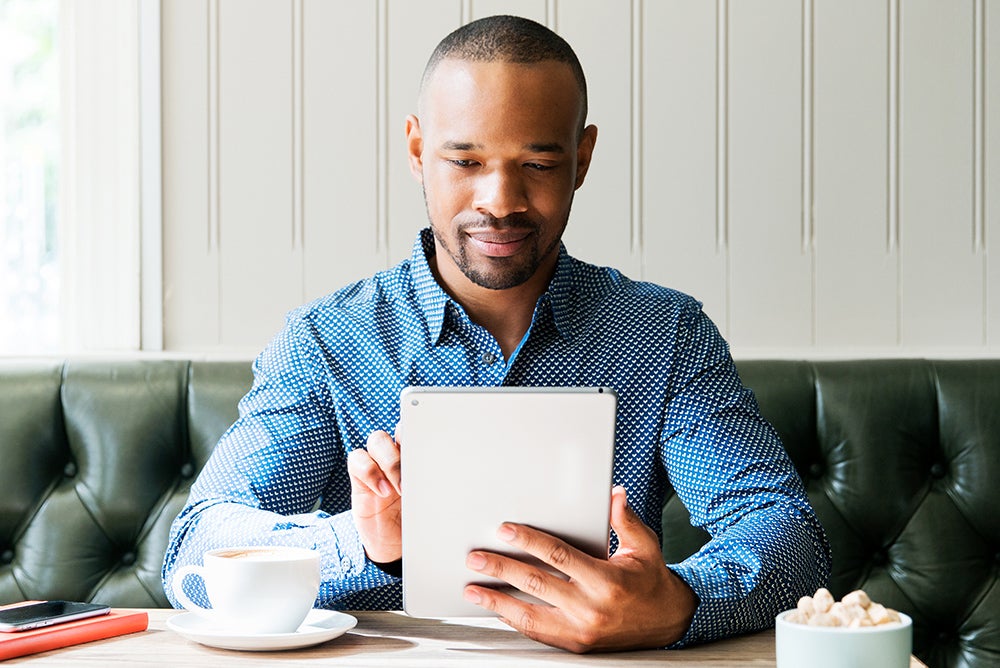
Editor's Note: This is the third and final of a three-part series offering insights into what healthcare marketers need to know to make informed decisions about using data to drive media campaigns, whom to get it from, and how to use it responsibly. Click here to view Part 1. Click here to view Part 2.
Consumer data has completely transformed how marketing and advertising get done, granting the ability to find who you need with fewer wasted dollars. But the use of personally identifiable health information (PII) is highly restricted, except in cases where you have consent. Given how difficult it is to get informed consent from a large number of individuals, most consumer data that we buy or use is modeled data. But caution: not all audience models are the same.
Using data attributes to accurately target the right consumer audience
Audience models are developed based on seed data. Data providers study a group of ‘seed’ people, then project out their observed attributes and behaviors to identify similar people in the greater population. This could include analyzing a number of different demographic variables, compared to millions of encounters, to predict consumers at risk for various service categories and encounter types within the next year. The bigger and more robust the seed data, the more reliable the model. The more reliable the model, the better you can target in-market consumers with the likelihood to have a specific condition and/or actively searching for or consuming condition-relevant content.
Some data suppliers use medical claims data to seed their databases, which can be extremely robust and include real historical diagnosis, treatment, and comorbidity information. Then, they model out this data to find other contextual audience targeting based on factors such as gender, age, online activity and engagement, health head of household (HHH), propensity to need services, and payer mix. For example, if the consumer model finds that commercially insured, digitally savvy men with an average income over $120K and a family history of heart disease have a higher propensity to be diagnosed with a form of cardiovascular disease, then a health system could use this model to identify high-value individuals with a propensity to seek care (and invest in their health) for their unified heart and vascular digital campaign strategy.
Another example: Let’s say the segment you’re interested in includes individuals who’ve consumed diabetes content. They are most likely to be motivated content seekers; caregivers or symptomatic but not yet (or newly) diagnosed; and having an issue or complication. Conversely, those who are living with diabetes long-term are probably not actively consuming diabetes content. If you’re looking for the total diabetes population, contextual data alone is unlikely to get you there. But if you’re a provider looking for the newly diagnosed, you can be confident that contextual data will get you to the right audience.
Finding the balance between scale, accuracy, and maturity
There’s a constant tradeoff in the world of data between scale and accuracy. The more you model out the seed data, the less accurate the output is going to be. Using the prior example, let’s say you start with adults with diabetes in your seed data. You then use machine learning to understand the various attributes shared by these individuals and create a model based on these characteristics. The model is then used to find consumers who look like your seeds. The first group identified is going to have extreme fidelity to the model. But this decreases as you bring more individuals into the segment.
So, the bigger the scale, the lower the accuracy. You need to decide where you want to land on that spectrum. Base it on your objective. If you’re launching a diabetes awareness campaign, you want your message broadcast far and wide. In this case, opt for scale. However, if you’re implementing a bottom-of-the-funnel conversion campaign, you want accuracy to ensure an efficient time-to- and cost-per-conversion.
And then there’s the factor of maturity, which can be either a good or bad thing, depending on how the model works. Some models are static and get stale. Others, that rely on machine learning and probabilistic inference, get smarter with time. When making your decision about an audience segment, finding out if the model it’s using is blooming or deteriorating with age will determine your ability to have the greatest health audience reach and positive clinical impact.
Final thought
Modeled data lets healthcare marketers find the audiences they need without having to delve into highly restricted PII. The more robust seed data you can utilize, the more reliable your model will be, helping you target (and talk to) the people you’re trying to reach. Just know that all data comes with compromises, based on scale, accuracy, and the age of the data.
Want to start from the beginning? We’ve created a comprehensive Insight Guide that you can download here. And, when you’re ready to explore how WebMD Ignite’s tailored Activation solutions can align with your organization's goals and drive tangible results, let's talk.