5 Best Practices in Healthcare Propensity Modeling
July 19, 2019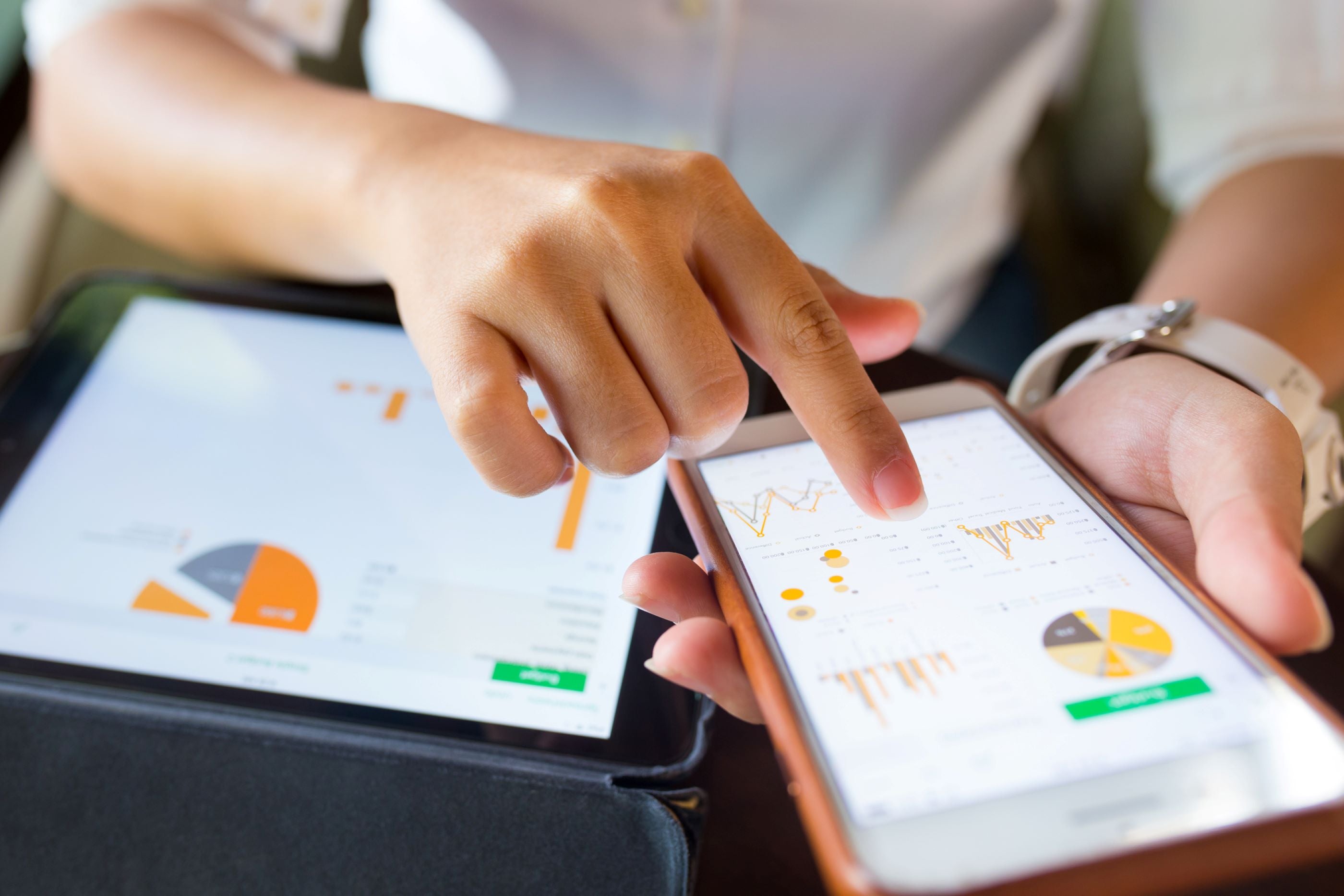
Categories: health systems, healthcare organizations
Editor's Note: This blog was published prior to the transition to WebMD Ignite.
The healthcare industry greatly impacts the growth of big data, but many healthcare systems find themselves lagging behind: They are data-rich yet analytics-poor. Big data offers opportunities for hospitals and health systems to better understand patient populations and behavior, ultimately leading to improved health outcomes.
But, as we’ve written about previously, it’s not enough to simply have the information. What’s critical for healthcare marketers is figuring out what they’re going to do with the information – and how to make data actionable. Enter propensity modeling.
What is propensity modeling?
By definition, propensity modeling, a subset of predictive modeling, is a family of multivariate statistical analyses used to optimize the prediction or likelihood of a specific event to occur.
In healthcare, propensity modeling involves using health analytics to identify the best prospects for targeted marketing efforts. Roughly translated, it’s a statistical approach that considers many variables at the same time, deriving insights from patterns and relationships in the data and using that information to create optimized and strategic marketing campaigns.
Healthcare marketers may use a set of diagnostic, visit history, sociodemographic, socioeconomic, lifestyle, and interest variables (below) to predict the likelihood a patient is, for example, a good candidate for hip replacement surgery.
But the million-dollar question is: With so many variables (and so much data) in play, how can healthcare marketers ensure they are effectively leveraging propensity and other predictive modeling tactics?
There are several critical factors that health systems should take into consideration when using propensity modeling to guide their marketing strategy:
Five best practices in healthcare propensity modeling
- Define your target or goal
- Use the best available data
- Use multiple data sources and the most appropriate analytics
- Ensure data is thoroughly vetted
- Deploy validated analytics and follow-up testing
1. Define your target or goal
Knowing exactly what your target is for any marketing campaign is critical. Ask yourself a few questions. Is this a general or a specific campaign? Do I want to target all possible prospects for a prostate or mammography screening campaign or focus on a very specific procedure, such a bariatric surgery?
In addition, take it one step further and identify what your primary purpose is. Are you looking for hand-raisers (i.e., campaign responders) or converters (i.e., convert to patients; have the procedure)? You also want to integrate the scope of the campaign. For example, a cross-sell initiative will be different than an acquisition focus.
- Cross-sell, Upsell, Retention: These models identify which patients are likely to respond to a diagnostic-specific marketing campaign (where “patients” typically defines individuals with a clinically-based interaction within a specified timeframe and exist in your current patient database).
- Acquisition and Re-Acquisition: These models identify which non-patients (consumers or past patients) in the target market geo footprint are most likely to respond to a diagnostic-specific marketing campaign.
The better-defined your targets and goals, the greater the likelihood you will select the right model for your needs – and therefore an increased likelihood you will be successful in meeting your objectives. Specifically, ensure the model was built using the level of detail that supports your primary purpose.
Key Takeaway: Clearly define your targets and goals. An “operational definition” is one that includes a specific measurement metric. For example, “all bariatric surgery prospects must have one or more diagnostic codes within the past three years indicating they are overweight or obese.” Additionally, ensure you have access to – and select from – multiple model types so as to employ analytics specific to a scenario: a consumer acquisition model is not intended for the same audience, nor goals, as a patient upsell model.
2. Use the best data
What is good data? The “best” data is both recent and “clean.” Data, whether it be consumer data from outside vendors or patient and physician data sourced from internal databases (EHR, HCRM, Credentialing System, etc.), should be as recent, and matched, cleaned, scrubbed, etc., as possible.
Find out when your data sources were last updated and confirm the standard update schedule. Also, ask for data summaries (audits, population reports, etc.) to ascertain depth, breadth, and fill rate of data elements. In addition, make sure to inquire about data cleansing and matching processes, as well as how outliers and disparate cases are handled.
Finally, whenever possible, use data that include standardized recodes. These are variables/fields that are clean and free of outliers that most vendors have available.
Key Takeaway: Garbage in – garbage out. For any marketing – or analytics – efforts, using dirty data will compromise your objective. Do what is in your control to use optimized and standardized data.
3. Use multiple data sources and most appropriate analytics
As mentioned earlier, if you have a well-defined target and goal, you are already optimizing your marketing efforts. The next step in this process is making sure you use the best analytics available for your efforts.
For example, many clients use standardized socio-demographic and socio-economic segmentation schemes for creating prospect lists and messaging. This is a standard as well as suggested practice.
To enhance this method, consider first using model scores as well as additional preselects and filters (such as segmentation methodologies) in your list creation process so that the target list is even more specific. The following is a suggested set of steps for a cardio screening list creation for younger males to be targeted for initial cardio screening and education:
- Pull the top 10-20% of scored records from a cardiac health model
- Include only males in the 35 to 54-year-old age groups
- Exclude any patients that have had a cardio screening in the last year
- Apply additional filters as necessary
Key Takeaway: Whether you use a combination of modeled and scored data, campaign response data, preselects and filters, and/or geo-based techniques to create your lists, always use the best analytics to meet your goal. An additional tip is to review your “best” performing past campaigns (converters, not just hand-raisers), and investigate what went into the creation of those lists!
4. Ensure data are vetted and validated by clinical/academic experts
In addition to the above, health systems seeking out propensity modeling vendors should inquire about testing/validation and whether models/techniques are vetted by clinical and academic audiences. In healthcare, it is critical that data, modeling, analytics, and targeted marketing are based on current empirical and best practices. For example, do you know that colon cancer rates are rising sharply for millennials but declining among the traditional 50+ demographic?
Make sure the diagnostic event groups (i.e., individuals who have received/experienced hip replacement, bariatric surgery, etc. in the past and thus the group upon which the model is based) are vetted by Physician Clinical Boards to ensure the validity of the clinical (ICD 9 and 10) and other codes utilized. Furthermore, before creating models, the diagnostic event groups should also be validated against empirical sources and best current practices.
Key Takeaway: Propensity modeling, as is the case with any advanced analytics, requires subject matter expertise. It is important to ensure the clinical inputs and statistical approaches applied are sound – or, minimally, are regularly reviewed by reputable, third-parties and that a variety of testing approaches are employed during the creation and maintenance of the models.
5. Deploy validated analytics and follow-up testing
Predictive modeling is most effective and efficient when employed in an ongoing lifecycle. Track, measure, and analyze the effect of the models on the overall success of your campaigns. Use this reflection to gain insights that you can use to improve your next campaign. Propensity modeling becomes a lifecycle when you use follow-up testing to better define your targets and goals.
Once the analytics (models) are deployed, a few follow-up validation practices are suggested:
- Utilize testing (e.g., messaging, creative)
- Profile and segment campaign responders, as well as campaign converters. Often, the profiles are different
- Track your ROMI (return on modeling investment) by models and response segments. Pay special attention to high dollar areas such as direct mail
In addition, go back and review that the deployed analytics:
- Are aligned with current or near-future campaigns
- Fit business rules and service delivery structure
- Are based on higher performing past campaigns
Key Takeaway: Employ testing and post-deployment analytics. Look for follow-up results that allow you to optimize your marketing efforts. Eliminate as much leakage as possible (e.g., are you including prospects that should not be targeted for the specific campaign?). Pay special attention to the most successful campaigns – the follow-up analyses will provide you with specifics about your best responders and converters.
Final thoughts
With the abundance of big data available in the healthcare space comes the opportunity to leverage that data to better understand patient populations and drive better health outcomes.
Propensity modeling, and additional predictive modeling and analytics, allows healthcare marketers to derive insights from patterns and correlations in data, using that information to pinpoint optimal targets, with statistical precision, and determine the consumers and patients most likely to respond to optimized targeted marketing campaigns.
By taking into consideration the above best practices, hospitals and health systems can better understand how to compare solutions and find the right platform to meet their organizations’ specific needs.